Artificial Intelligence
Neural Networks Achieve Human-Like Language Generalization
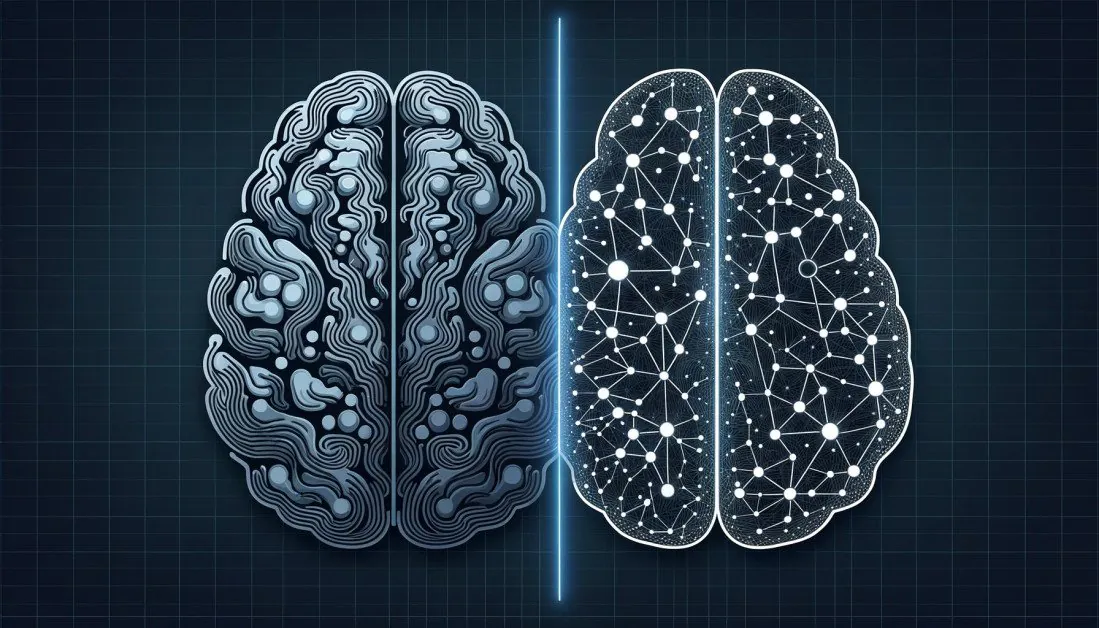
In the ever-evolving world of artificial intelligence (AI), scientists have recently heralded a significant milestone. They've crafted a neural network that exhibits a human-like proficiency in language generalization. This groundbreaking development is not just a step, but a giant leap towards bridging the gap between human cognition and AI capabilities.
As we navigate further into the realm of AI, the ability for these systems to understand and apply language in varied contexts, much like humans, becomes paramount. This recent achievement offers a promising glimpse into a future where the interaction between man and machine feels more organic and intuitive than ever before.
Comparing with Existing Models
The world of AI is no stranger to models that can process and respond to language. However, the novelty of this recent development lies in its heightened capacity for language generalization. When pitted against established models, such as those underlying popular chatbots, this new neural network displayed a superior ability to fold newly learned words into its existing lexicon and use them in unfamiliar contexts.
While today's best AI models, like ChatGPT, can hold their own in many conversational scenarios, they still fall short when it comes to the seamless integration of new linguistic information. This new neural network, on the other hand, brings us closer to a reality where machines can comprehend and communicate with the nuance and adaptability of a human.
Understanding Systematic Generalization
At the heart of this achievement lies the concept of systematic generalization. It's what enables humans to effortlessly adapt and use newly acquired words in diverse settings. For instance, once we comprehend the term ‘photobomb,' we instinctively know how to use it in various situations, whether it's “photobombing twice” or “photobombing during a Zoom call.” Similarly, understanding a sentence structure like “the cat chases the dog” allows us to easily grasp its inverse: “the dog chases the cat.”
Yet, this intrinsic human ability has been a challenging frontier for AI. Traditional neural networks, which have been the backbone of artificial intelligence research, don't naturally possess this skill. They grapple with incorporating a new word unless they've been extensively trained with multiple samples of that word in context. This limitation has been a subject of debate among AI researchers for decades, sparking discussions about the viability of neural networks as a true reflection of human cognitive processes.
The Study in Detail
To delve deeper into the capabilities of neural networks and their potential for language generalization, a comprehensive study was conducted. The research was not limited to machines; 25 human participants were intricately involved, serving as a benchmark for the AI's performance.
The experiment utilized a pseudo-language, a constructed set of words that were unfamiliar to the participants. This ensured that the participants were truly learning these terms for the first time, providing a clean slate for testing generalization. This pseudo-language comprised two distinct categories of words. The ‘primitive' category featured words like ‘dax,' ‘wif,' and ‘lug,' which symbolized basic actions akin to ‘skip' or ‘jump'. On the other hand, the more abstract ‘function' words, such as ‘blicket', ‘kiki', and ‘fep', laid down rules for the application and combination of these primitive terms, leading to sequences like ‘jump three times' or ‘skip backwards'.
A visual element was also introduced into the training process. Each primitive word was associated with a circle of a specific color. For instance, a red circle might represent ‘dax', while a blue one signified ‘lug'. Participants were then shown combinations of primitive and function words, accompanied by patterns of colored circles that depicted the outcomes of applying the functions to the primitives. An example would be the pairing of the phrase ‘dax fep' with three red circles, illustrating that ‘fep' is an abstract rule to repeat an action thrice.
To gauge the understanding and systematic generalization abilities of the participants, they were presented with intricate combinations of the primitive and function words. They were then tasked with determining the correct color and number of circles, further arranging them in the appropriate sequence.
Implications and Expert Opinions
The results of this study are not just another increment in the annals of AI research; they represent a paradigm shift. The neural network's performance, which closely mirrored human-like systematic generalization, has stirred excitement and intrigue among scholars and industry experts.
Dr. Paul Smolensky, a renowned cognitive scientist with a specialization in language at Johns Hopkins University, hailed this as a “breakthrough in the ability to train networks to be systematic.” His statement underscores the magnitude of this achievement. If neural networks can be trained to generalize systematically, they can potentially revolutionize numerous applications, from chatbots to virtual assistants and beyond.
Yet, this development is more than just a technological advancement. It touches upon a longstanding debate in the AI community: Can neural networks truly serve as an accurate model of human cognition? For nearly four decades, this question has seen AI researchers at loggerheads. While some believed in the potential of neural networks to emulate human-like thought processes, others remained skeptical due to their inherent limitations, especially in the realm of language generalization.
This study, with its promising results, nudges the scales in favor of optimism. As Brenden Lake, a cognitive computational scientist at New York University and co-author of the study, pointed out, neural networks might have struggled in the past, but with the right approach, they can indeed be molded to reflect facets of human cognition.
Towards a Future of Seamless Human-Machine Synergy
The journey of AI, from its nascent stages to its current prowess, has been marked by continuous evolution and breakthroughs. This recent achievement in training neural networks to generalize language systematically is yet another testament to the limitless potential of AI. As we stand at this juncture, it's essential to recognize the broader implications of such advancements. We are inching closer to a future where machines not only understand our words but also grasp the nuances and contexts, fostering a more seamless and intuitive human-machine interaction.
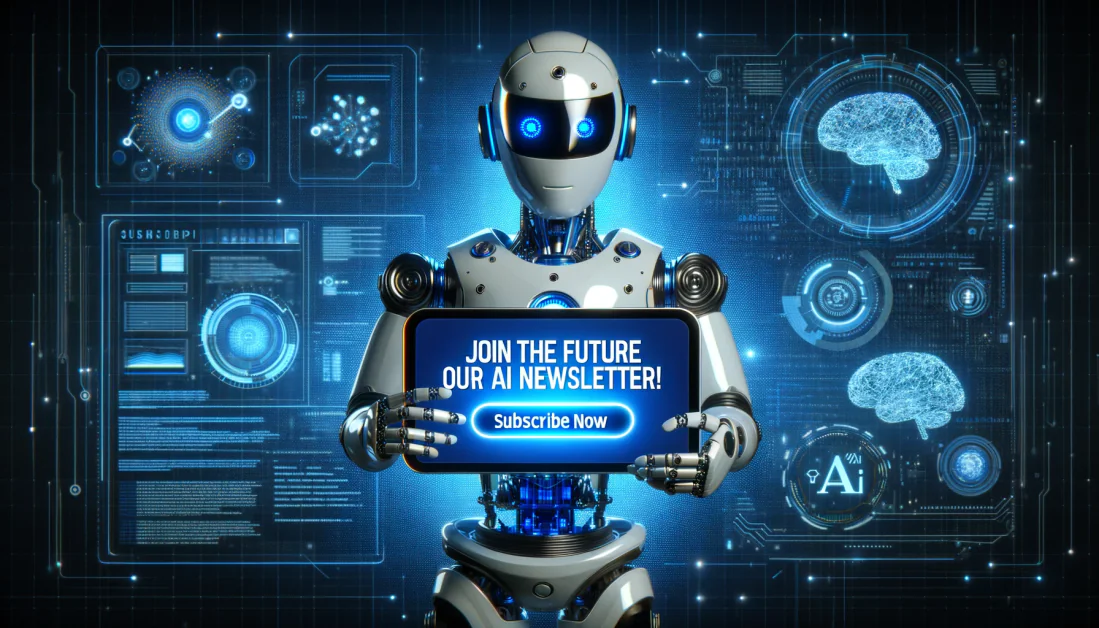
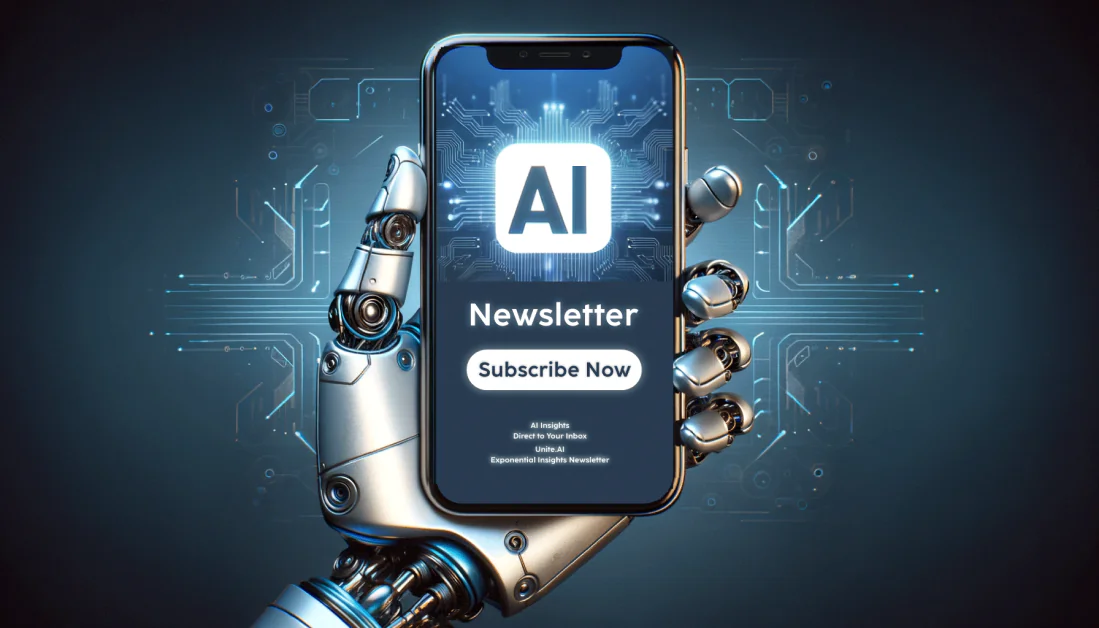