Artificial General Intelligence
Connecting the Dots: Unravelling OpenAI’s Alleged Q-Star Model
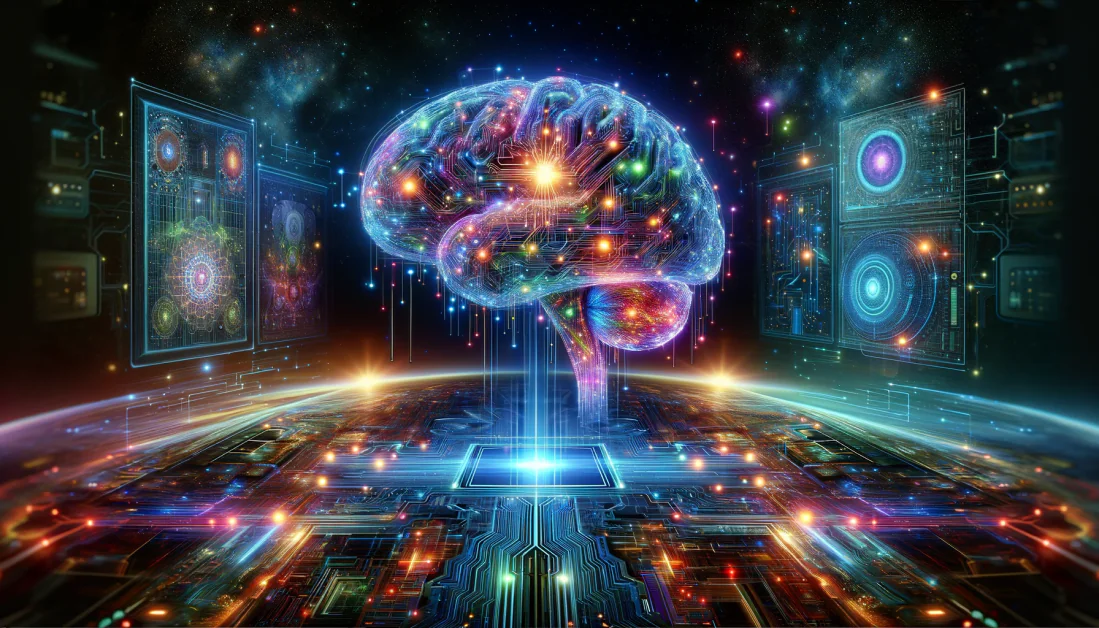
Recently, there has been considerable speculation within the AI community surrounding OpenAI's alleged project, Q-star. Despite the limited information available about this mysterious initiative, it is said to mark a significant step toward achieving artificial general intelligence—a level of intelligence that either matches or surpasses human capabilities. While much of the discussion has focused on the potential negative consequences of this development for humanity, there has been relatively little effort dedicated to uncovering the nature of Q-star and the potential technological advantages it may bring. In this article, I will take an exploratory approach, attempting to unravel this project primarily from its name, which I believe provides sufficient information to glean insights about it.
Background of Mystery
It all began when the board of governors at OpenAI suddenly ousted Sam Altman, the CEO, and co-founder. Although Altman was reinstated later, questions persist about the events. Some see it as a power struggle, while others attribute it to Altman's focus on other ventures like Worldcoin. However, the plot thickens as Reuters reports that a secretive project called Q-star might be the primary reason for the drama. As per Reuters, Q-Star marks a substantial step towards OpenAI's AGI objective, a matter of concern conveyed to the board of governors by OpenAI's workers. The emergence of this news has sparked a flood of speculations and concerns.
Building Blocks of the Puzzle
In this section, I have introduced some building blocks that will help us to unravel this mystery.
- Q Learning: Reinforcement learning is a type of machine learning where computers learn by interacting with their environment, receiving feedback in the form of rewards or penalties. Q Learning is a specific method within reinforcement learning that helps computers make decisions by learning the quality (Q-value) of different actions in different situations. It's widely used in scenarios like game-playing and robotics, allowing computers to learn optimal decision-making through a process of trial and error.
- A-star Search: A-star is a search algorithm which help computers explore possibilities and find the best solution to solve a problem. The algorithm is particularly notable for its efficiency in finding the shortest path from a starting point to a goal in a graph or grid. Its key strength lies in smartly weighing the cost of reaching a node against the estimated cost of reaching the overall goal. As a result, A-star is extensively used in addressing challenges related to pathfinding and optimization.
- AlphaZero: AlphaZero, an advanced AI system from DeepMind, combines Q-learning and search (i.e., Monte Carlo Tree Search) for strategic planning in board games like chess and Go. It learns optimal strategies through self-play, guided by a neural network for moves and position evaluation. The Monte Carlo Tree Search (MCTS) algorithm balances exploration and exploitation in exploring game possibilities. AlphaZero's iterative self-play, learning, and search process leads to continuous improvement, enabling superhuman performance and victories over human champions, demonstrating its effectiveness in strategic planning and problem-solving.
- Language Models: Large language models (LLMs), like GPT-3, are a form of AI designed for comprehending and generating human-like text. They undergo training on extensive and diverse internet data, covering a broad spectrum of topics and writing styles. The standout feature of LLMs is their ability to predict the next word in a sequence, known as language modelling. The goal is to impart an understanding of how words and phrases interconnect, allowing the model to produce coherent and contextually relevant text. The extensive training makes LLMs proficient at understanding grammar, semantics, and even nuanced aspects of language use. Once trained, these language models can be fine-tuned for specific tasks or applications, making them versatile tools for natural language processing, chatbots, content generation, and more.
- Artificial General intelligence: Artificial General Intelligence (AGI) is a type of artificial intelligence with the capacity to understand, learn, and execute tasks spanning diverse domains at a level that matches or exceeds human cognitive abilities. In contrast to narrow or specialized AI, AGI possesses the ability to autonomously adapt, reason, and learn without being confined to specific tasks. AGI empowers AI systems to showcase independent decision-making, problem-solving, and creative thinking, mirroring human intelligence. Essentially, AGI embodies the idea of a machine capable of undertaking any intellectual task performed by humans, highlighting versatility and adaptability across various domains.
Key Limitations of LLMs in Achieving AGI
Large Language Models (LLMs) have limitations in achieving Artificial General Intelligence (AGI). While adept at processing and generating text based on learned patterns from vast data, they struggle to understand the real world, hindering effective knowledge use. AGI requires common sense reasoning and planning abilities for handling everyday situations, which LLMs find challenging. Despite producing seemingly correct responses, they lack the ability to systematically solve complex problems, such as mathematical ones.
New studies indicate that LLMs can mimic any computation like a universal computer but are constrained by the need for extensive external memory. Increasing data is crucial for improving LLMs, but it demands significant computational resources and energy, unlike the energy-efficient human brain. This poses challenges for making LLMs widely available and scalable for AGI. Recent research suggests that simply adding more data doesn't always improve performance, prompting the question of what else to focus on in the journey towards AGI.
Connecting Dots
Many AI experts believe that the challenges with Large Language Models (LLMs) come from their main focus on predicting the next word. This limits their understanding of language nuances, reasoning, and planning. To deal with this, researchers like Yann LeCun suggest trying different training methods. They propose that LLMs should actively plan for predicting words, not just the next token.
The idea of “Q-star,” similar to AlphaZero's strategy, may involve instructing LLMs to actively plan for token prediction, not just predicting the next word. This brings structured reasoning and planning into the language model, going beyond the usual focus on predicting the next token. By using planning strategies inspired by AlphaZero, LLMs can better understand language nuances, improve reasoning, and enhance planning, addressing limitations of regular LLM training methods.
Such an integration sets up a flexible framework for representing and manipulating knowledge, helping the system adapt to new information and tasks. This adaptability can be crucial for Artificial General Intelligence (AGI), which needs to handle various tasks and domains with different requirements.
AGI needs common sense, and training LLMs to reason can equip them with a comprehensive understanding of the world. Also, training LLMs like AlphaZero can help them learn abstract knowledge, improving transfer learning and generalization across different situations, contributing to AGI's strong performance.
Besides the project's name, support for this idea comes from a Reuters' report, highlighting the Q-star's ability to solve specific mathematical and reasoning problems successfully.
The Bottom Line
Q-Star, OpenAI's secretive project, is making waves in AI, aiming for intelligence beyond humans. Amidst the talk about its potential risks, this article digs into the puzzle, connecting dots from Q-learning to AlphaZero and Large Language Models (LLMs).
We think “Q-star” means a smart fusion of learning and search, giving LLMs a boost in planning and reasoning. With Reuters stating that it can tackle tricky mathematical and reasoning problems, it suggests a major advance. This calls for taking a closer look at where AI learning might be heading in the future.
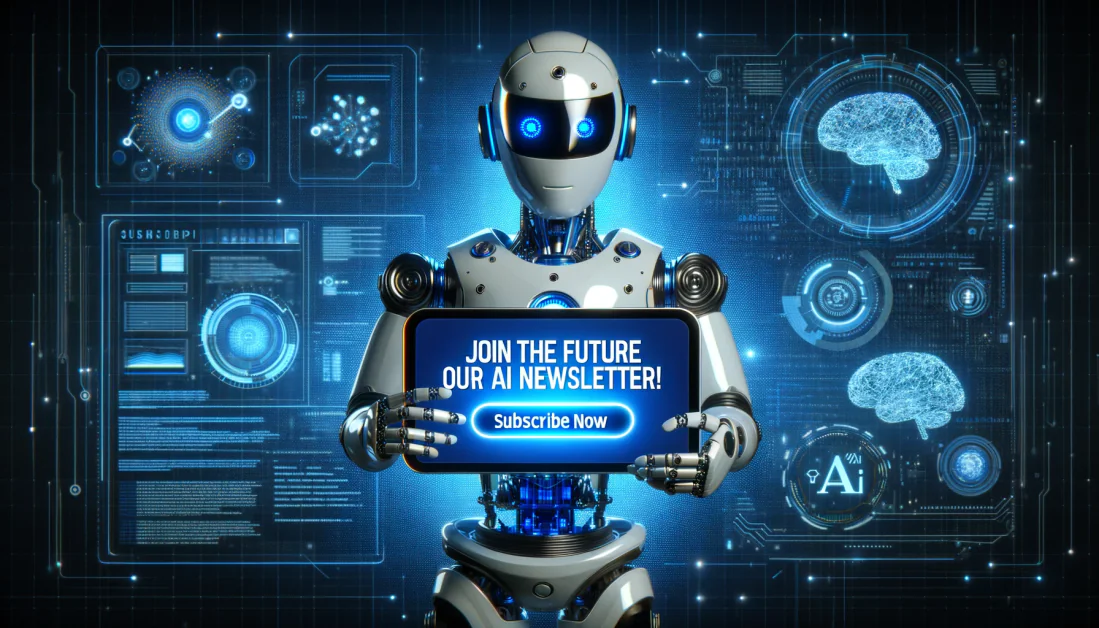
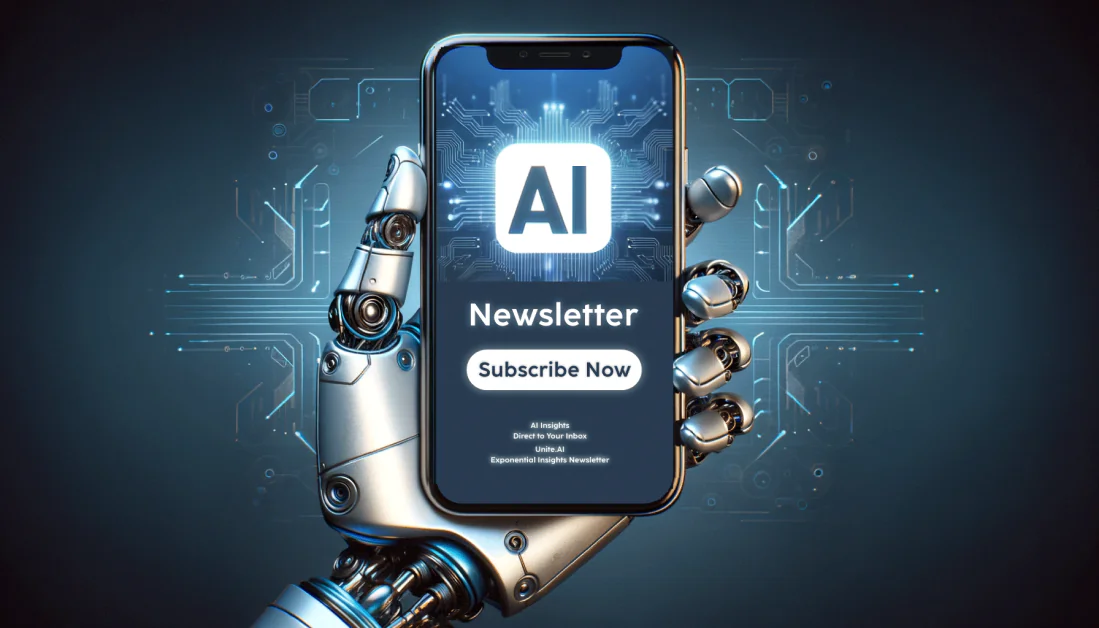