Is Your Call Center Stuck in the Stone Age? Modernizing QA for the AI Era
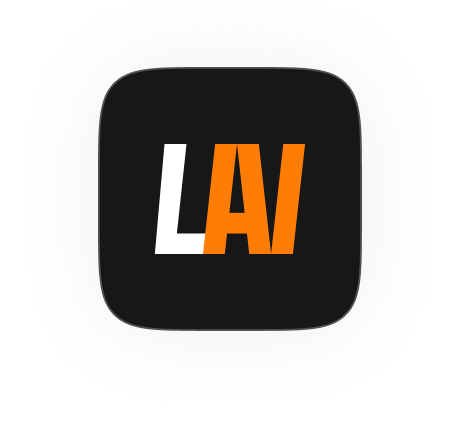
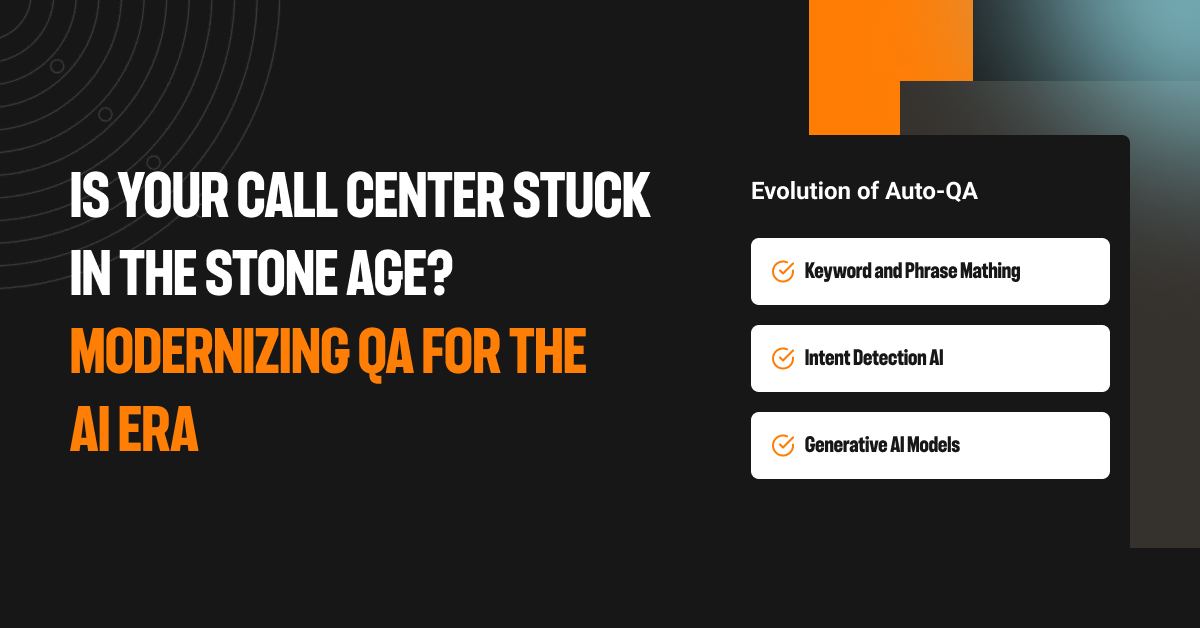
In the relentless pursuit of exceptional customer experiences, optimizing call center operations remains a top priority for smart organizations.
At the heart of this task lies quality assurance (QA), a vital process that ensures that all customer interactions are handled impeccably.
As technology continues to evolve, so too must the way contact centers approach QA.
In this article, we examine the way QA technology has changed (or hasn’t changed) over the years and how AI-powered QA systems represent the next step in the evolution of call center QA.
Generation 0: Sample-based quality monitoring
Before purpose-built QA systems arrived on the scene, contact center managers used sample-based quality monitoring systems that allowed them to only monitor 1% to 5% of all conversations.
This approach is a very manual, labor-intensive process. To get the insights they need, QA teams spend hours sifting through silences and non-coachable parts of customer interactions — which isn’t exactly the best use of time.
While sample-based quality monitoring is better than not doing QA at all, it leaves much to be desired in today’s fast-paced world.
Generation 1: Keyword and sentiment-based systems
In the early days of call center QA technology, keyword and sentiment-based systems were first on the scene. These first-generation systems provided a rudimentary evaluation, primarily focusing on high-level aspects of interactions.
While they could detect elements like proper greetings, empathy, and closing language, their evaluation was surface-level at best. They could offer a sentiment score and identify a handful of predefined topics which required a lot of tedious, manual intervention to maintain. But they were incapable of the comprehensive analysis required for customer service excellence. These systems lacked the depth required to truly assess the quality of contact center interactions.
Despite their limitations, keyword and sentiment-based systems served as a stepping stone in the evolution of QA technology. They laid the groundwork for more advanced systems to follow, demonstrating the potential for automation in the QA process.
However, as call center operations grew in complexity and customer expectations soared, it became evident that a more sophisticated approach was needed. The shortcomings of these systems became increasingly apparent, prompting the development of the next generation of QA technology.
Generation 2: Phrase-based systems
The second generation brought about phrase-based systems, promising automation for simple yes-no questions. These systems aimed to streamline QA processes by automating the evaluation of basic criteria such as agent verification or the retrieval of customer information.
However, the capabilities of these systems remained limited. They could only handle a small set of predetermined questions, often around 10 at most. Consequently, the reliability of their scores came into question, and their applicability to diverse scenarios was severely restricted.
While phrase-based systems represented an improvement over their predecessors, they still fell short of providing a comprehensive solution to QA challenges. Their rigid framework and limited scope hindered their effectiveness in accurately evaluating the nuances of customer interactions.
For example, such a system might be trained to look for the phrase “let me help you” and grade those interactions glowingly. But there’s a huge difference between an agent saying, “Sure, let me help you!” and “You won’t let me help you, so there’s no point in talking.” These systems can’t tell the difference between such nuances.
As such, QA managers and contact center leaders found themselves grappling with the same issues they had faced with keyword and sentiment-based systems. It was clear that a more advanced approach was needed to meet the evolving demands of the modern call center.
Generation 3: Intent-Based Systems
Enter the third generation: AI-powered systems.
Representing the pinnacle of modern QA technology, these systems revolutionize the call evaluation process. Unlike their predecessors, AI-powered systems can analyze entire conversations across each interaction, providing a holistic understanding of every conversation.
Crucially, these systems have the flexibility to adapt to the unique requirements of different businesses. For example, the QA rubric for a retail giant like Walmart would differ significantly from that of a financial institution like Bank of America. AI-powered systems can tailor their evaluations accordingly, ensuring relevance and accuracy out of the gate.
Moreover, AI-powered systems leverage advanced algorithms and machine learning techniques to continuously improve their performance over time. With each interaction analyzed, these systems become smarter and more adept at identifying patterns and trends. This iterative process of learning ensures that AI-powered systems remain relevant and effective in the ever-changing landscape of customer interactions. QA managers and contact center leaders can rely on these systems to provide accurate and actionable insights, enabling them to drive improvements in agent performance and customer satisfaction.
Generation 4: QA-GPT, your new, human-like QA auditor
At the forefront of this AI revolution stands QA-GPT, your new, human-like QA auditor. It’s the industry’s first generative AI large language model (LLM) that evaluates every conversation across every customer channel — your ticket out of the Stone Age and into the future.
Leveraging cutting-edge technology, QA-GPT can evaluate nearly 100% of QA rubrics with near-human accuracy. Its capabilities are mind-blowing as it continues to improve with each interaction. Like ChatGPT, QA-GPT understands what each question on the rubric actually means. For example, if the question is Did the agent greet the customer?, QA-GPT knows what’s being asked and proceeds to score each interaction with no further input.
That’s compared to an intent-based model, which needs to be trained to recognize what “greeting the customer” means by providing a few sample phrases. QA-GPT, on the other hand, learns the intent behind phrases and can match even completely different phrases and words that weren’t trained with the same intent.
Some questions — Did the agent reduce effort for the customer? — are impossible for an intent-based model to score. In this example, there’s no universal intent that can capture “reducing the customer’s effort” because that varies across every interaction.
This is precisely where QA-GPT shines. This leading-edge technology automatically understands what “reducing the customer’s effort” means without any further input, and can score conversations directly.
What’s more, setting up QA-GPT is a breeze, requiring minimal time and resources. Gone are the days of opaque scoring systems; QA-GPT provides clear evidence for every score, fostering transparency and accountability.
Powered by a proprietary LLM, QA-GPT continuously learns and evolves based on your own data, ensuring its relevance and effectiveness in the ever-changing landscape of customer interactions. That’s compared to other AI systems that use the slow OpenAI API, which is not trained on contact center data.
QA-GPT offers unparalleled flexibility, allowing QA managers to customize evaluation criteria based on their unique business needs. Whether it's specific industry regulations, company policies, or customer preferences, QA-GPT can adapt to accommodate any requirements. This level of customization ensures that QA evaluations are tailored to each organization's specific goals and objectives.
With QA-GPT, QA managers and contact center leaders can unlock new levels of efficiency, accuracy, and customer satisfaction. Here’s how:
- QA-GPT automatically evaluates over 80% of scorecards, understanding language nuances and generating reasoning for every auto-score — directly from the conversation data.
- QA-GPT is trained on your company’s call center data and automatically and consistently learns and improves, with a proprietary LLM that goes beyond keywords and phrases to understand language nuances.
- QA-GPT doesn’t require manual reviewing and listening to entire conversations to validate AI evaluations, unlike other AI solutions on the market.
Ready to accelerate contact center QA?
The evolution of QA technology mirrors the evolution of customer service itself. From humble beginnings marked by keyword and sentiment-based systems to the cutting-edge AI-powered solutions of today, the journey has been transformative.
For QA managers and contact center leaders, it’s imperative to embrace this evolution and equip teams with the tools they need to thrive in the AI era.
By modernizing QA processes and adopting QA-GPT, teams can unlock new levels of efficiency, accuracy, and customer satisfaction — much to the delight of your business and your bottom line.
The future of call center QA is here. Are you ready to embrace it?
For more information on QA-GPT, check this out.
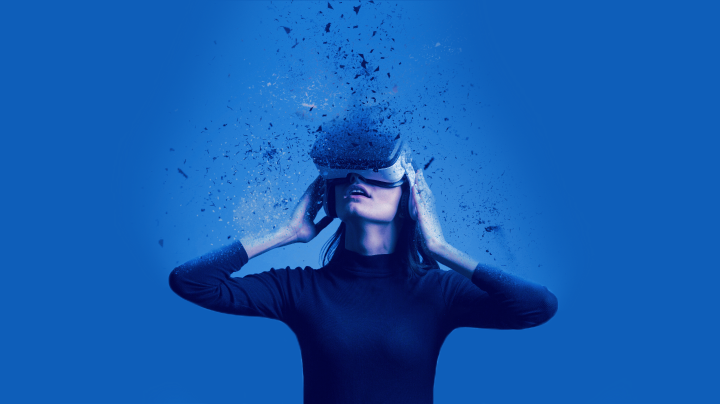
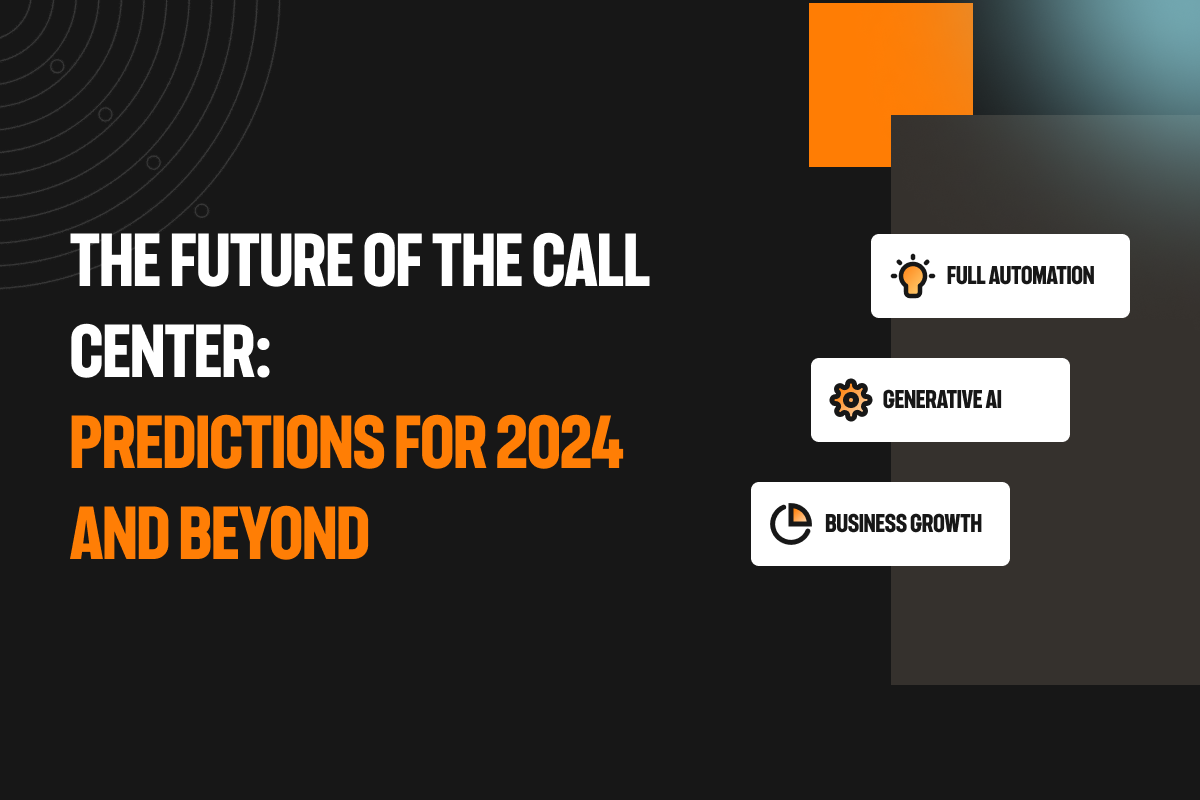
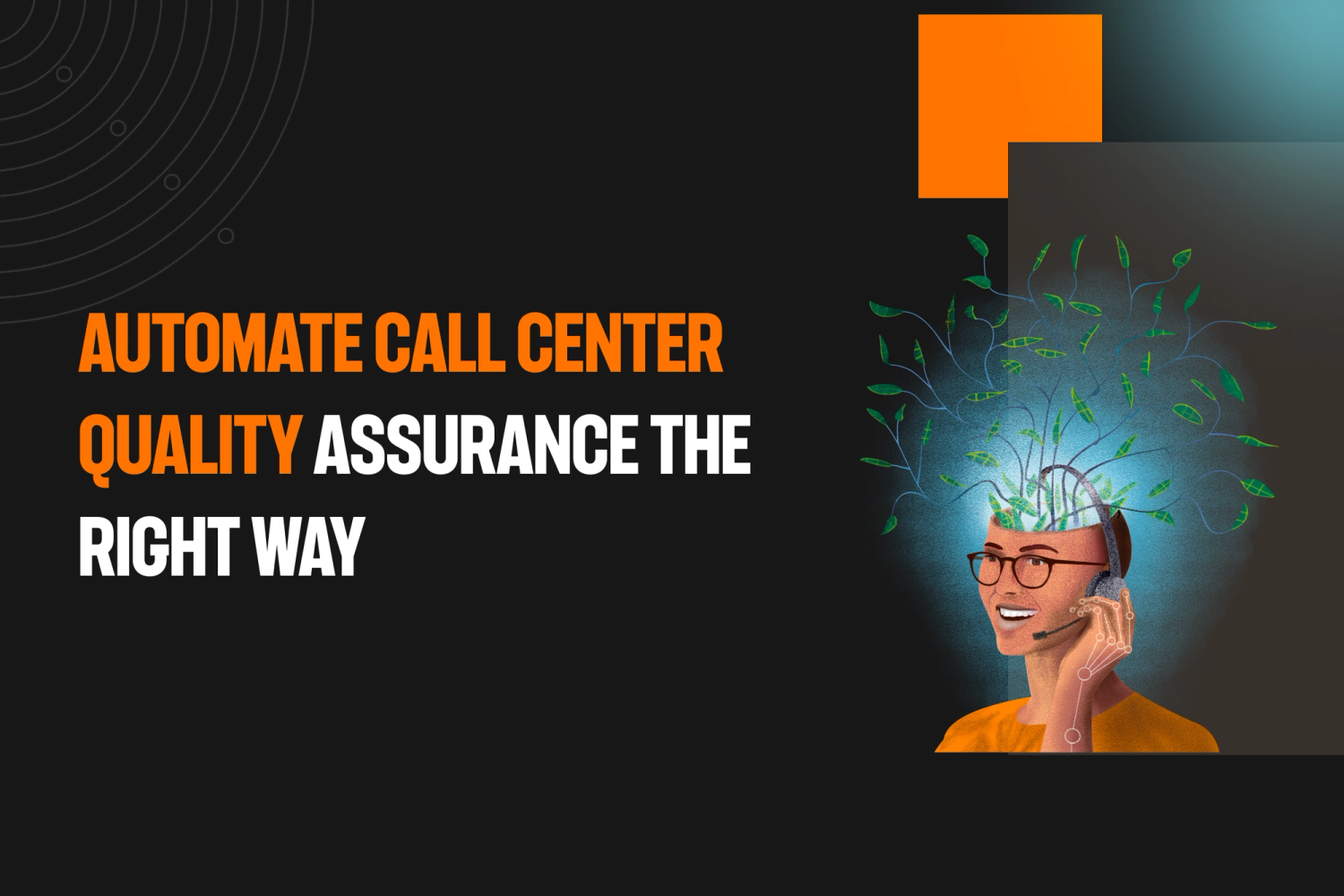