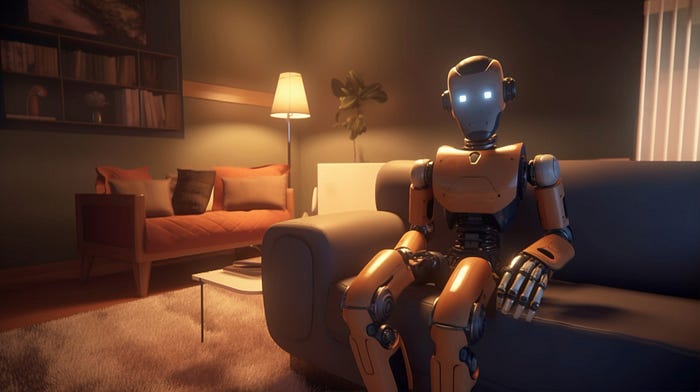
How do artificial intelligence, machine learning, deep learning and neural networks relate to each other?
Last Updated on August 22, 2023 by Editorial Team
Author(s): AlishaS
Originally published on Towards AI.
Machine Learning vs. AI vs. Deep Learning vs. Neural Networks: What’s the Difference?
The rapid evolution of technology is molding our everyday existence as businesses turn more and more to sophisticated algorithms for efficiency. Amidst this backdrop, we often hear buzzwords like artificial intelligence (AI), machine learning (ML), deep learning, and neural networks thrown around almost interchangeably. But don’t be fooled — while these terms might appear interchangeable, they each encompass distinct concepts and technologies that contribute to the growing realm of intelligent machines.
Steering through this intricate landscape requires a keen grasp of the subtle differences that set these technologies apart. This article is your compass, designed to unravel the complexities and shed light on the individualities that differentiate these tech trends. Let’s dive deeper and uncover what sets them apart →
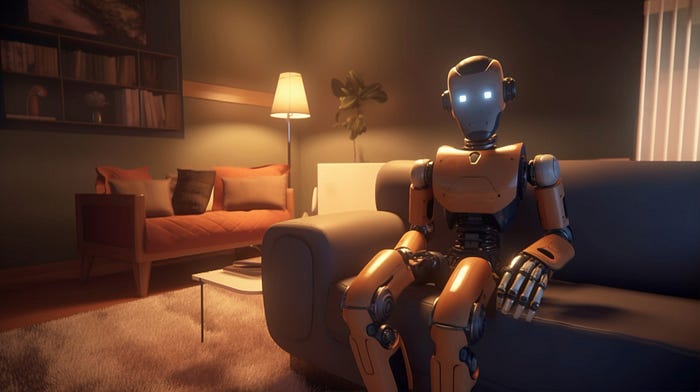
Imagine a sequence of Russian nesting dolls, each fitting neatly within the other. Similarly, the relationship between artificial intelligence (AI), machine learning (ML), deep learning, and neural networks can be visualized as a hierarchy, where each term encapsulates and builds upon the one before it.
- Artificial Intelligence (AI): At the highest level, we have Artificial Intelligence (AI), representing machines that mimic human intelligence, including problem-solving and learning. AI handles complex tasks like facial recognition, speech analysis, and decision-making through predictions and automation. Within AI, there are categories like Artificial Narrow Intelligence (ANI), which focuses on specific tasks; Artificial General Intelligence (AGI), reaching human-like abilities; and the theoretical concept of Artificial Super Intelligence (ASI), surpassing human capabilities.
- Machine Learning (ML): Next, machine learning takes the spotlight. ML employs algorithms that learn patterns from data to perform tasks without explicit programming. It powers applications like chatbots, recommendation systems, and fraud detection, residing as a subset within the broader realm of AI.
- Deep Learning: Delving further, we encounter Deep Learning, a subset of machine learning that streamlines feature extraction, excelling with extensive datasets. This technology employs artificial neural networks, essentially its building blocks, with interconnected layers mirroring the human brain’s structure. This approach benefits complex tasks like virtual assistants and fraud detection due to its error-driven learning capability.
- Neural Network: Deep learning leverages artificial neural networks, which are the building blocks of this technology. These networks are characterized by their layers of interconnected nodes, simulating the structure of the human brain. Deep learning proves advantageous in complex tasks like virtual assistants and fraud detection due to its ability to automatically learn from errors.
Now, let’s explore key distinctions to gain a clearer grasp of these AI components, all while remembering the essence of artificial intelligence.
What Exactly is Artificial Intelligence (AI)?
AI refers to the incredible capability of machines to mimic various functions of the human mind. This includes tasks like thinking, predicting outcomes, and even managing intricate tasks without requiring human involvement. This technology can be divided into three main types:
- Artificial Narrow Intelligence (ANI): Sometimes called “weak” AI, ANI is exceptionally adept at handling specific tasks with a high degree of skill. For example, it’s exceptionally proficient at playing chess or accurately identifying faces in photographs.
- Artificial General Intelligence (AGI): On the flip side, there’s what we term “strong” AI or AGI. This kind of AI possesses cognitive abilities akin to human thinking. It can execute tasks at a level that’s comparable to human capabilities. Essentially, it’s like having a machine that can think and learn much in the same way we humans do.
- Artificial Super Intelligence (ASI): Now, envision AI that goes beyond human intelligence and abilities — that’s ASI. At this stage, AI becomes somewhat of a theoretical notion, still awaiting full realization. Think of it as picturing a machine that can outperform humans in nearly every intellectual pursuit.
So, that’s a glimpse into the realm of AI — a realm where machines aren’t just tools but companions on our journey into the future. From mastering specific tasks to emulating our general intellect, and who knows, perhaps one day even pushing the boundaries of what we believed to be achievable — that’s the captivating narrative of Artificial Intelligence.
Deep Learning vs. Machine Learning
In the world of artificial intelligence, we often encounter two terms: Deep Learning and Machine Learning. Although they might seem similar, they have distinct ways of working with data and learning. To simplify, Deep Learning is a specialized part of Machine Learning, differing in how they process information.
Think of these as various tools in an AI toolbox. Deep Learning shines with unstructured data, automating the extraction of meaningful patterns. This makes it great for complex tasks. Remember, Deep Learning and Machine Learning aren’t rivals; Deep Learning is just one method within the larger realm of Machine Learning.

Here’s what sets Deep Learning apart:
- Structure of Algorithms: Deep Learning relies on complex artificial neural networks that consist of multiple layers. These layers function similarly to the interconnected neurons found in the human brain. They process data hierarchically, systematically revealing intricate patterns that might be hidden within the information.
- Human Involvement: In contrast to traditional Machine Learning methods, Deep Learning minimizes the need for direct human intervention in the process of feature extraction. It possesses the capability to learn from its own errors, progressively enhancing its performance over time without constant manual adjustments by humans.
- Demand for Data: Deep Learning systems exhibit a substantial appetite for data, which is more pronounced compared to standard Machine Learning algorithms. This elevated requirement is due to the intricate architecture of neural networks, which necessitates a copious amount of data to derive accurate and meaningful insights.
- So, when we delve into the world of AI and its various approaches, remember that Deep Learning and Machine Learning aren’t adversaries but rather collaborators, each with its own strengths and applications.
Deep Learning vs. Neural Networks
The term “deep” in deep learning signifies the depth of layers within a neural network. A neural network with more than three layers, including input and output layers, qualifies as a deep learning algorithm. These deep neural networks can be feed-forward, moving from input to output, or trained using back-propagation, adjusting based on calculated errors. Explore the different types of deep neural networks and interview questions on deep learning.
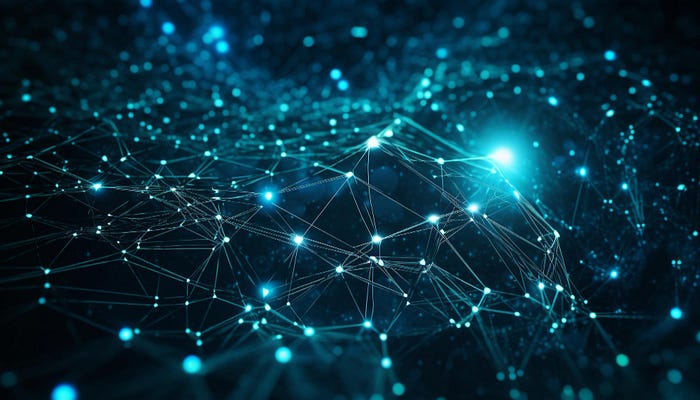
Key differences between deep learning and neural networks include:
- Complexity: Deep learning networks are notably more intricate compared to traditional neural networks. This complexity arises from their utilization of multiple layers stacked together.
- Efficiency: When it comes to efficiency and overall effectiveness, deep learning systems have proven to surpass traditional neural networks. They can handle and process complex data more adeptly.
- Components: Deep learning units demand significant computational resources, such as powerful GPUs and ample RAM. In contrast, neural networks are composed of fundamental elements like neurons, connections, and propagation functions.
- Training Time: The training process for deep learning networks is lengthier due to their intricate nature, requiring more time to converge. On the other hand, neural networks, being simpler, demand less training time to reach convergence.
AI’s Role in the Equation
Artificial Intelligence (AI) acts as the overarching idea that encompasses the fields of machine learning, deep learning, and neural networks. AI and machine learning are closely related but maintain their individual identities. Machine learning operates within the realm of AI, and deep learning, in its turn, falls under the umbrella of machine learning.
Let’s delve deeper into these distinctions:
- Artificial Intelligence vs. Machine Learning: Imagine AI as the broader concept of machines acting smart, while machine learning is a specific method within AI. Machine learning deals with algorithms that learn patterns from data, allowing machines to accomplish tasks without explicit programming.
- Artificial Intelligence vs. Neural Networks: AI involves crafting machines that simulate human thinking. On the other hand, neural networks are intricate structures inspired by the human brain, comprising interconnected artificial neurons. These networks help machines recognize patterns and learn from data.
- Artificial Intelligence vs. Deep Learning: Picture AI as the grand scheme of creating smart machines. Inside that, deep learning is a specialized part of machine learning. It relies on complex algorithms and vast datasets to teach models intricate patterns. In essence, AI covers a broader scope while deep learning is a powerful technique within it.
Conclusion
In the dynamic and ever-changing world of technology, it’s crucial to have a clear grasp of the differences between artificial intelligence, machine learning, deep learning, and neural networks. Think of them as layers in a hierarchy — AI being the overarching concept, with machine learning, deep learning, and neural networks nested within.
Mastery of these concepts isn’t just about staying savvy in the digital realm; it’s about unlocking your own potential. Don’t let the intricate terminology deter you. Instead, keep in mind that these technologies collaborate harmoniously, shaping innovative solutions that mold our reality. So, as you embark on your journey through AI, machine learning, deep learning, and neural networks, remember that they’re interconnected pieces propelling the advancement of technology in our era.
Join thousands of data leaders on the AI newsletter. Join over 80,000 subscribers and keep up to date with the latest developments in AI. From research to projects and ideas. If you are building an AI startup, an AI-related product, or a service, we invite you to consider becoming a sponsor.
Published via Towards AI