Computers Are Learning to Smell
AI could revolutionize our understanding of one of the most mysterious human senses.
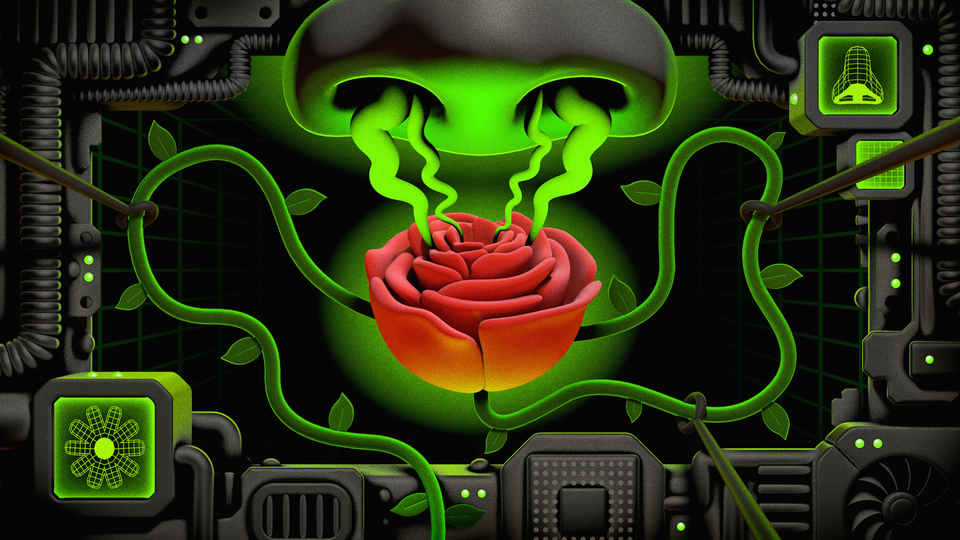

Listen to this article
Listen to more stories on hark
You know the smell of warm, buttered popcorn. A crisp autumn day. The pungent, somewhat sweet scent that precedes rain. But could you begin to describe these aromas in detail? Or compare them? Your nose has some 400 olfactory receptors that do the work of translating the world’s estimated 40 billion odorous molecules into an even higher number of distinct scents your brain can understand. Yet although children are taught that grass is green and pigmented by chlorophyll, they rarely learn to describe the smell of a freshly cut lawn, let alone the ozone before a storm. The ability to express our sense of smell, in part because we’ve ignored it, eludes most of us.
Humans are not alone in this limitation. We have invented machines that can “see” and “hear”: Audio was first recorded and played back in 1877, and the first moving image followed a year later. A musical note is defined by its pitch, a single number, and computers represent a color with three numbers—the red, green, and blue (RGB) values that correspond to the types of color-receiving cells in our eyes. A song is a sequence of sounds, and an image, a map of pixels. But there has never been a machine that can flawlessly detect, store, and reproduce odors.
Scientists are working to change that. At the end of August, researchers published a paper presenting a model that can describe a molecule’s scent as well as, or even better than, a person (at least in limited trials). The computer program does so by placing molecules on a sort of odor map, where flowery smells are closer together than to, say, rotten ones. By quantitatively organizing odors, the research could mark a significant advance in enhancing our understanding of human perception. As it has already done for the study of vision and language, AI may be auguring a revolution in the study of this more enigmatic human sense.
“The last time we digitized a human sense was a generation ago,” Alex Wiltschko, a neuroscientist and a co-author of the paper, told me. “These opportunities don’t come around that often.” Computers can’t quite smell yet, but this research is a big step toward that goal, which Wiltschko began pursuing at Google Research and is now the focus of his start-up, Osmo. “People have been trying to predict smell from chemical structure for a long time,” Hiroaki Matsunami, a molecular biologist at Duke who studies olfaction and was not involved with the study, told me. “This is the best at this point in order to do that task. In that sense, it’s a great advance.”
Machine-learning algorithms require a huge amount of data to function, and the only information available for a scent comes from notoriously unreliable human noses and brains. (Even slight tweaks to a molecule can make a sweet, banana-scented compound reek of vomit; mysterious changes to your nose and brain, as many unfortunately learned from developing COVID-19, can make coffee smell of sewage.) Wiltschko and his team set out to identify and curate a set of roughly 5,000 molecules and associated odor descriptions (“alcoholic,” “fishy,” “smoky,” and so on) from researchers in the flavor and fragrance industries, then fed that data to a type of algorithm called a graph neural network, which was able to represent each molecule’s atoms and chemical bonds in a sort of internal diagram. The resulting program can, given a molecule’s structure, predict how it will smell as a combination of the existing odor labels.
Testing those predictions’ accuracy presented a whole other challenge. The team had to train a new, independent group of people to smell and label a new set of molecules that the program had never analyzed. “People are really bad at [describing scents] when they walk off the street,” Joel Mainland, a neuroscientist at the Monell Chemical Senses Center, in Philadelphia, who helped conduct the training for the study, told me. “If you train them for a couple hours, they get pretty good, pretty fast.”
Over five one-hour sessions, participants were given different substances associated with one of 55 different odors, such as kombucha (“fermented”), a crayon (“waxy”), or a green-apple Jolly Rancher (“apple”), to learn a reference point for each label. Participants then took a test in which they had to describe the smell of 20 common molecules (vanillin is vanilla-scented; carvone is minty), and then retook the test to make sure their judgments were consistent, Emily Mayhew, a food scientist at Michigan State University and co-author of the study, told me. Everybody who passed could help validate the algorithm.
The researchers curated a set of molecules that was highly distinct from the set used to train the program, then had participants smell and describe all of the new molecules with various labels, each rated from zero to five (hypothetically, a lemon might receive a five for “citrus,” a two for “fruity,” and a zero for “smoky.”). The average of all those ratings became the benchmark against which to compare the computer. “If you take two people and you ask them to describe a smell, they will often disagree,” Mainland said. But an average of several smell-trained people is “pretty stable.”
Overall, the AI model “smelled” a bit more accurately than the people participating in the research. The program provides “a really powerful demonstration that some key aspects of our odor perception are shared,” Sandeep Robert Datta, a neurobiologist at Harvard who did not conduct the research but is an informal adviser to Osmo, told me. Exactly what two people think a lemon smells like varies, but most will agree a lemon and an orange both smell of citrus, and an apple does not.
Then there’s the study’s map. Every molecule, and in turn its odor, can be numerically represented in a mathematical space that the authors call a “principal odor map.” It provides insight into not just the relation between structure and smell but also the way our brain organizes odors, Wiltschko told me: Floral scents are in one section of the map, meaty ones in another; lavender is closer to jasmine on the map than it is to a beefy aroma.
Datta cautioned that he would not describe the odor map as principal so much as perceptual. “It does a beautiful job of capturing the relationship between chemistry and perception,” he said. But it doesn’t take into account all the steps—from receptors in our nose to the cerebral cortex in our brain—that occur as a molecule is turned into chemical signals that are then transformed into verbal descriptions of a smell. And the map isn’t like RGB values in that it doesn’t describe basic components that can make any smell—although it does “suggest to us that RGB [for smell] is possible.” The computer model’s perceptual odor map is an “extraordinarily important proof of concept,” he added, and provides crucial insights into how the brain appears to organize smells. For instance, you might assume certain categories of smell—citrus and smoky, for instance—are entirely separate, Datta said. But the odor map suggests that paths connect even these disparate scents.
The model is just the first in many advances needed to digitize scent. “It still lacks some of the important aspects of smell,” Matsunami told me, which the paper’s authors readily admit. Their program cannot predict how molecules smell in combination, and most natural odors are the results of very complex mixtures. It also wasn’t designed to take into account odor concentration, which can change not just the degree but also the quality of a smell (the molecule MMB, for instance, gives off a pleasant odor in small doses and is added to household cleaners, but in high concentrations it helps make cat urine smell like cat urine.) That the model also predicts a smell only on average makes it unclear how well the program would do in real-world settings, given people’s individual perceptions, Datta said. Even though the research is like the “Manhattan Project for categorizing odor qualities relative to physical, chemical parameters,” Richard Doty, the director of the Smell and Taste Center at the University of Pennsylvania, who was not involved with the study, told me, it’s unclear to him how much further the model can bring our understanding of smell given how complex our nose is. “I don’t know where it leads us.”
Still, future research could tackle some of these problems, Wiltschko argues, and fine-tune the map itself. The number of dimensions in the map, for instance, is arbitrarily set to optimize the computer program; changes in the training data might improve the model as well. And studying other parts of our olfactory system, such as receptors in our nose or neural pathways to the brain, will likely also help reveal more about how and through what stages the human body processes various smells. One day, a set of programs that can translate the structure, concentration, and mixture of molecules into a smell, paired with a chemical sensor, could truly realize digital olfaction.
Even without proper Smell-o-Vision, it is shocking, in a sense, that a computer model removed from the facts of human embodiment—a program has no nose, olfactory bulb, or brain—can reliably predict how something smells. “The paper implicitly advances the argument that you don’t need to understand the brain in order to understand smell perception,” Datta said. The research reflects a new, AI-inflected scientific understanding that seems to be popping up everywhere—using chatbots to study the human brain’s language network, or using deep-learning algorithms to fold proteins. It is an understanding rooted not in observation of the world so much as that of data: prediction without intuition.