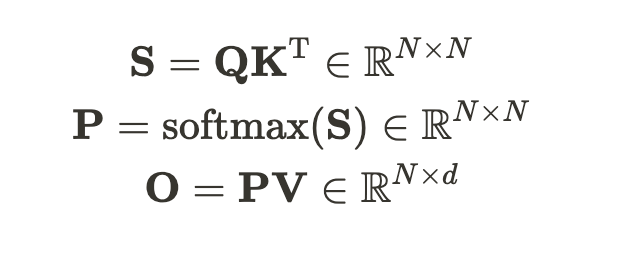
Unveiling FlashAttention-2
Last Updated on January 3, 2024 by Editorial Team
Author(s): Florian
Originally published on Towards AI.
The demand for new scenarios, such as long document queries and story writing, has resulted in an increase in the context length of large language models. For example, GPT-4 has a context length of 32k, and Anthropic’s Claude has a context length of 100k. However, expanding the context length of the Transformer poses a major challenge due to the quadratic runtime and memory requirements of the attention layer, which is at the core of these models.
To address this challenge, FlashAttention[1] is introduced as an attention mechanism that speeds up attention and reduces its memory footprint without any approximation. While FlashAttention is already 2 to 4 times faster than standard attention, there is still potential for further improvement.
In contrast, FlashAttention-2[2], proposed in July 2023, is twice as fast as FlashAttention and can even achieve 10 times the speed of PyTorch’s standard attention.
This article will explore the principles and improvements of FlashAttention-2 to provide readers with a deeper understanding of this algorithm.
Given Q, K, and V of the input sequence, we need to calculate the attention output tensor O:
where 𝑁 is the sequence length and 𝑑 is the head dimension, softmax is applied row-wise. To improve clarity in the explanation, we omit… Read the full blog for free on Medium.
Join thousands of data leaders on the AI newsletter. Join over 80,000 subscribers and keep up to date with the latest developments in AI. From research to projects and ideas. If you are building an AI startup, an AI-related product, or a service, we invite you to consider becoming a sponsor.
Published via Towards AI